A new method for evaluating neural networks may improve the identification of brain disorders
Applicable to the clinical diagnosis of brain and psychiatric diseases, it has been developed by Vicente Pallarés, Andrea Insabato, Anna Sanjuán and Matthieu Gilson, researchers of the Center for Brain and Cognition, led by ICREA researcher Gustavo Deco, with international participation, recently published in the journal NeuroImage.
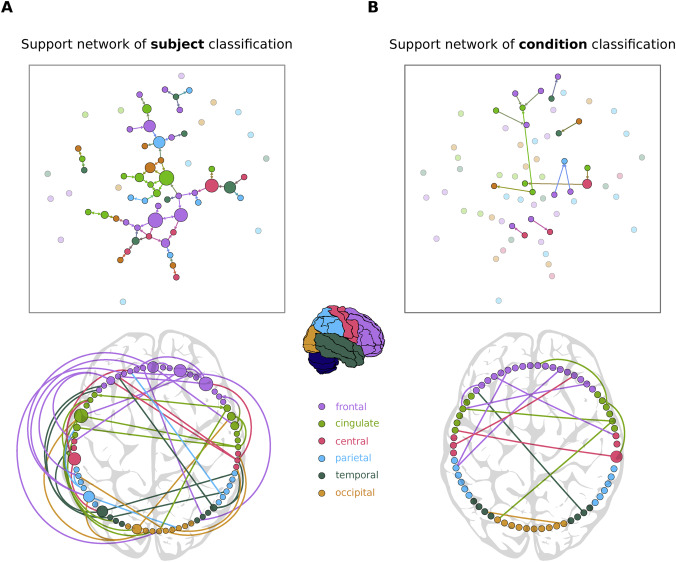
Functional magnetic resonance imaging (fMRI) measures the amount of oxygen in the blood in specific areas of the brain and is an indirect test of neuronal activity. fMRI uses radiofrequency waves and a powerful magnetic field to obtain highly detailed images of the brain. This technique has been used in the clinical diagnosis of neurological and psychiatric diseases, and alterations in brain functionality constitute clinical signs of different brain and behavioural disorders.
However, the study of brain connectivity based on images from functional magnetic resonance imaging is sometimes limited by the fact that these measures can vary from one session to another depending on the experimental or study conditions, which endangers their reproducibility and reliability on an individual level. In order to be able to apply the results of research in real clinical practice, often with few recording sessions, it becomes crucial to clarify the diagnostic value of fMRI in each case.
The key to a sound prediction is the use of effective connectivity
The goal of a study published in open access on 24 May in the advanced online edition of the journal NeuroImage was to set a new reliable standard for the extraction of biomarkers based on fMRI, verifying that information of different brain states, at rest or active, does not get mixed with the identity of each person. The results indicate that the key to a sound prediction is the use of effective connectivity (EC) instead of functional connectivity (FC) and suitable machine learning techniques.
“The results obtained establish solid foundations for applications adapted to individual cognitive states in clinical diagnosis”
The study was conducted by Vicente Pallarés, Andrea Insabato, Anna Sanjuán and Matthieu Gilson, researchers with the Center for Brain and Cognition, which is led by Gustavo Deco, ICREA research professor at the Department of Information and Communication Technologies (DTIC) at UPF, involving centres in Europe and the United States.
Functional connectivity (FC) of the brain in resting state indicates the areas of the brain whose activity is correlated, whereas effective connectivity (EC) between different brain areas indicates how some areas have a direct influence over others. In this regard, effective connectivity describes real (or causal) interactions between the areas and is measured using a dynamic model. This paper considers that effective connectivity supports better performance than functional connectivity in the classification of brain state.
A reliable standard for the extraction of biomarkers of brain activity
The new method proposed by the authors provides information on the communication between brain areas according to brain activity or state and consists of two parts. In the first part, the estimation of the effective connectivity of the whole brain is estimated with suitable machine learning tools to control variability from session to session. “We have demonstrated how machine learning tools, such as MLR (Multinomial Logistic Regression), allow quantifying topological ‘orthogonality’ between biomarkers, in addition to extracting them efficiently”, the authors note.
The researchers’ idea was to compare the values of EC and FC with generalization capability through fMRI sessions at resting state compared to other states. “Our study shows that EC-based biomarkers are very robust to session-to-session variability (much more than with FC) and can be obtained based on a limited number of sessions, namely, 4-5 recording sessions (5 minutes each) to classify 40 or more other sessions”, the researchers suggest.
“On the basis of very few brain connections, our method proves to have excellent generalization capabilities for the identification of cognitive states and of participants in two sets of data, using only a few sessions as a reference”
In the second part of the method, the researchers predict the identity and condition of each participating individual -in resting state and performing a visual task- to verify that effective connectivity (EC) can be used as a biomarker for the two types of state. To do so, “we examine the topological distribution of the links of EC that give support to dual classification, which allows us to quantify the overlap between the two biomarkers”, the authors explain. This second part has sought to assess the generalization capability of EC for multivariate classification. “Our method, with very few brain connections, has been seen to have excellent generalization capabilities for identifying cognitive states and participants in two datasets, using only a few sessions as a reference”, they added.
In this study, we propose a theoretical framework to extract biomarkers associated with different modalities on the basis of fMRI data to enable quantifying the interference between biomarkers. The dual classification of the identity of the participants and the behavioural conditions goes beyond the previous studies that used functional connectivity as “fingerprint”, and so “our results establish solid foundations for applications adapted to cognitive states in clinical diagnosis”, suggest the authors of this research.
Reference work:Vicente Pallarés, Andrea Insabato, Ana Sanjuán, Simone Kühn, Dante Mantini, Gustavo Deco, Matthieu Gilson (2018), “Extracting orthogonal subject- and condition-specific signatures from fMRI data using whole-brain effective connectivity“, NeuroImage, vol. 178, September 2018, pp. 238-254, https://doi.org/10.1016/j.neuroimage.2018.04.070.