Gemma Boleda
5. Kaleidoscope
Linguistics and artificial intelligence: why not?
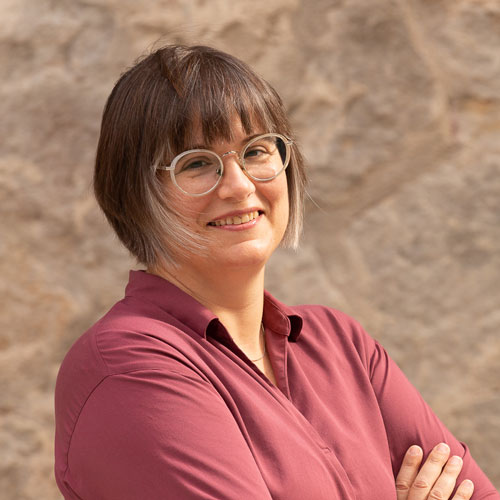
Gemma Boleda, ICREA Research Professor in the Department of Translation and Language Sciences and head of the COLT Research Group: Computational Linguistics and Linguistic Theory
When I discovered linguistics in the first year of my undergraduate programme in Spanish philology, it was love at first sight. I had finally found my calling! Since then, I have devoted myself to understanding how language works. Towards the end of the programme, I began to gravitate more towards quantitative and computational methods. I ended up doing a PhD in computational linguistics, a field that straddles linguistics and artificial intelligence.
What drew me to artificial intelligence was the possibility of analysing vast amounts of data, such as the millions of texts available on the Internet. Extracting systematic knowledge from such a large quantity of data cannot be done by hand; AI gave me tools to do it. In particular, I apply a method called machine learning, in which you feed a computer a large amount of data and ask it to extract patterns that enable it to perform a given task, such as translating a text from English to Catalan. To be able to perform such a complex task, the computer needs to acquire a great deal of linguistic knowledge; consequently, examining its behaviour can shed a lot of light on how language works. This methodology has been used, for instance, to investigate how words can have different meanings in different contexts, or to determine the genealogical relationships between languages.
But not only does AI have enormous potential for the scientific study of language, it is also the basis of the language technology we interact with on a daily basis: when we write messages on WhatsApp and it suggests words to us, when we use the AutoCorrect feature in Word, when we translate texts with Google Translate, and so on.
But not only does AI have enormous potential for the scientific study of language, it is also the basis of the language technology we interact with on a daily basis: when we write messages on WhatsApp and it suggests words to us, when we use the AutoCorrect feature in Word, when we translate texts with Google Translate, and so on.
Both the development of language technology and research in computational linguistics require knowledge of linguistics and artificial intelligence; but, in our education system, it is quite difficult to cover both fields. For example, half the students on the master’s programme in Theoretical and Applied Linguistics at UPF, where I teach, want to specialize in computational linguistics. The problem is that most have a background in ‘the arts’, and the gap between the training they need and the training they have received up to the master’s programme is huge. As a result, even though they are highly motivated, they have to invest a lot of additional time and effort to acquire the ‘scientific’ knowledge they need. And that, in turn, makes it difficult for them to contribute all their knowledge about language to technology or research. It is clearly a doubly missed opportunity.
This is just one of many examples of the problems caused by the strict division between science and the arts in our education system. Interdisciplinarity is often said to be key to 21st-century society; in the case of computational linguistics, that is crystal clear. Yet not only does our education system fail to encourage interdisciplinarity, it actively prevents it. When I was in secondary school, I liked maths and literature; but when I was 16, I had to choose one or the other. For those choosing their upper secondary school track this year, three decades later, the situation is essentially the same. The structures need to be made more flexible to allow the new generations to acquire more interdisciplinary training starting in secondary school; and we all need to build strong bridges between complementary disciplines and methods. Fortunately, many of us are already doing our part to build them.